Pursuing enhanced computational efficiency and precision, Dr. Kateryna Lemishko’s recent presentation at ICOPS 2024 introduced an innovative machine learning approach for estimating plasma reaction kinetic data. This study addresses the critical issue of limited availability of input data for plasma models, which traditionally depend on time-consuming experiments and first-principles calculations.
The Challenge
Plasma modelling necessitates a vast amount of kinetic data, including cross sections and rate coefficients, which are often scarce. This lack of data poses a significant challenge to the development of accurate plasma models, which are essential for numerous applications in computational chemistry and plasma physics.
The Solution
Dr. Lemishko’s research harnesses the power of machine learning to deliver fast and reasonably accurate estimations of plasma reaction rate coefficients. This innovative approach not only reduces the time required but also makes it possible to estimate kinetic data for a large number of reactions involving heavy particles.
Methodology
The machine learning model developed by Dr. Lemishko is trained on a comprehensive dataset comprising approximately 12,500 reactions with either two or three products. The input features for this model include a wide array of properties: masses, charges, standard enthalpy of formation, polarizability, dipole moments, ionisation energy, and species elemental composition.
To address the issue of missing values, dedicated machine learning models were employed to predict critical properties such as enthalpy of formation, polarizability, and dipole moments. This robust preprocessing step ensures the accuracy and reliability of the input data used to train the machine learning model.
Model Implementation and Performance
Several conventional machine learning models were evaluated during the study, including Ridge Regression, K-Nearest Neighbors Regression, Support Vector Regression (SVR), Random Forest, and Gradient Boosting. The best-performing models were then integrated into a voting regressor to enhance overall prediction accuracy.
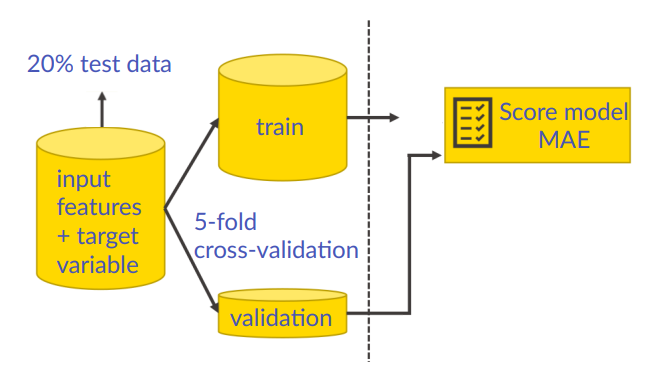
Figure 1. Visual representation of conventional machine learning model
The model was segmented based on reactant charge combinations and the number of products, with separate algorithms developed for exothermic and endothermic reactions. This segmentation approach allowed for more precise modelling of the different types of reactions.
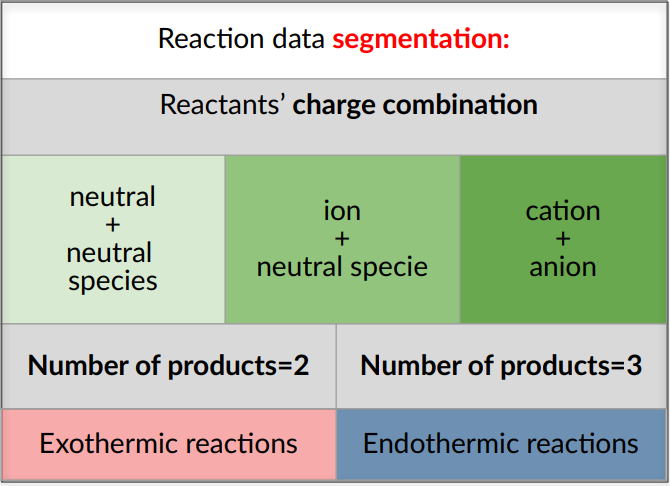
Figure 2. Visual representation of reaction data segmentation
The performance of the model was rigorously tested, yielding mean absolute errors (MAE) as low as 0.31 for specific exothermic reactions, demonstrating the efficacy of this approach.
Results
The machine learning estimator developed in this study demonstrated impressive performance across various reaction types:
- Exothermic Reactions: Achieved a mean absolute error of 0.31 for 746 test reactions.
- Endothermic Reactions: Achieved a mean absolute error of 0.84 for 175 test reactions.
- General Reactions: For example, in reactions like A+ + B– → C + D, the mean absolute error was found to be 1.15 for exothermic and 1.17 for endothermic reactions.
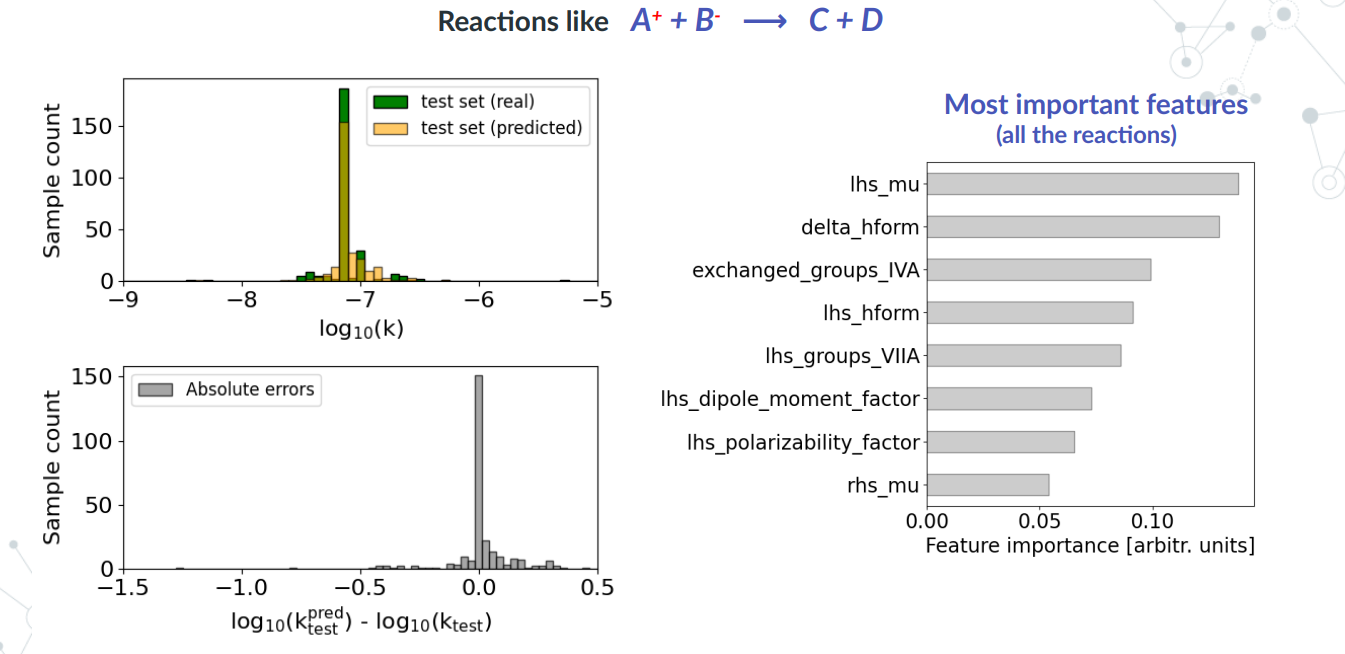
Figure 3. Graphical representation of result data for reactions like A+ + B– → C + D
These results underscore the model’s ability to accurately predict rate coefficients across a diverse set of plasma reactions, providing a valuable tool for researchers and practitioners in the field.
Conclusions
Dr. Lemishko’s machine learning-based algorithm represents a significant advancement in the estimation of plasma reaction kinetics. By deriving rate coefficients from a rich set of input features, this approach facilitates the rapid approximation of unknown plasma reaction rate constants at 300 K. This amazing approach accelerates the development of plasma models and exemplifies the transformative potential of machine learning in scientific research.
Key Takeaways
- Machine learning offers a fast and cost-effective alternative to traditional experimental methods for estimating plasma reaction kinetics.
- The approach significantly reduces the time required to obtain kinetic data for a large number of reactions.
- This innovation highlights the growing intersection of machine learning and computational chemistry, paving the way for future advancements in the field.
Dr. Kateryna Lemishko’s work underscores the importance of interdisciplinary approaches in tackling complex scientific problems and highlights the potential of machine learning to improve plasma science and beyond.